Advancing rare disease classification: exploring representation learning in low-data and heavy tail settings
Laure Ciernik — Hector Fellow Klaus-Robert Müller
This project seeks to advance rare disease classification using deep neural networks by addressing key challenges such as limited data and high heterogeneity. We will assess existing models and their representations, investigating how technical variations in medical data affect their characteristics. Additionally, we will utilize representation learning on large, unlabeled datasets, with an emphasis on DNA methylation data, to improve knowledge transfer from common to rare diseases. Finally, we will also focus on addressing label imbalance in model training, ensuring more accurate and well-calibrated disease predictions.
Accurate disease classification is crucial for timely diagnosis and tailored treatments. However, this becomes challenging with rare diseases due to limited data, high heterogeneity, and complexity. This project focuses on rare disease classification with deep neural networks. We will use models that project data into representation spaces that capture the semantic categories of diseases. Several aspects will be explored.
First, we will assess existing models and their learned representations, evaluating their characteristics and similarities and identifying desirable traits. Additionally, we aim to investigate how technical variations in medical data, such as data sources and patient characteristics known as batch effects, affect model representations and explore methods for mitigating them.
Secondly, we would like to use representation learning on large, unlabeled datasets to capture biological patterns across various conditions. This approach has been shown to facilitate knowledge transfer from common to rare diseases. Our primary focus will be on utilizing DNA methylation data, which, to the best of our knowledge, lacks models found for other data types like histopathology and single-cell RNA sequencing.
Finally, we will address label imbalance, which remains an issue even with good representations. Therefore, we will investigate training techniques for the downstream disease classification task that can handle this imbalance and produce well-calibrated predictions.
Representation learning in low-data and heavy tail settings.
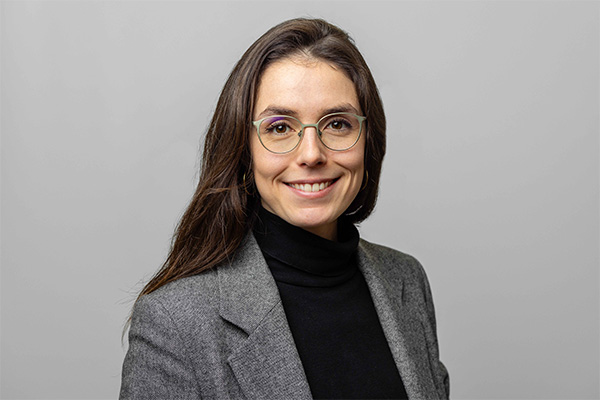
Laure Ciernik
Technische Universität BerlinSupervised by
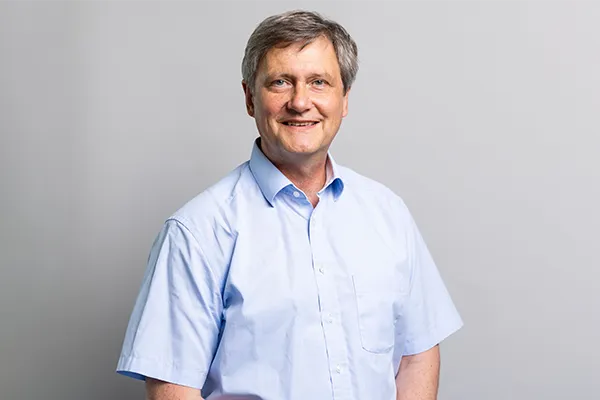
Klaus-Robert Müller
Informatics, Mathematics & PhysicsHector Fellow since 2023