Representation Learning and Causality: Theory, Practice, and Implications for Mechanistic Interpretability
Florent Draye — Hector Fellow Bernhard Schölkopf
My research interests lie at the intersection of representation learning, causality, and generative models. I aim to contribute to the development of methods that extract informative and interpretable features from high-dimensional datasets, with a focus on uncovering high-level causally related factors that describe meaningful semantics of the data. This, in turn, can help us gain deeper insights into the representations found within advanced generative models, particularly foundation models and LLMs, with the goal of improving their efficiency and safety.
Deep neural networks have demonstrated significant success across various tasks due to their ability to learn meaningful features from complex, high-dimensional data. However, their heavy reliance on large amounts of labelling to effectively learn these features restricts their applicability in unsupervised learning scenarios. Representation learning tries to transform these high-dimensional datasets into lower dimensional representations without supervision. By identifying the right space in which to perform reasoning and computation, it also enables the discovery of interpretable patterns and features within the data. In my PhD, I am working at the intersection of representation learning and causality. I am researching how to learn data representations that are both interpretable and controllable, focusing on identifying the appropriate high-level abstractions of the data on which to model causal structures.
In parallel, foundation models like large language models (LLMs) have recently gained significant attention. These neural networks, trained on massive datasets with billions of parameters, are often considered "black boxes" due to our lack of understanding of their underlying computational principles. Gaining a deeper understanding of the algorithms implemented by these neural networks is essential, not only for advancing scientific knowledge but also for improving their efficiency and safety. To address this challenge, I am integrating concepts from causality and representation learning to build a mechanistic understanding of the high-dimensional data representations found within these advanced generative models.
Part 1. Representation Learning / causal representation learning. Part 2. Building a mechanistic understanding of the high-dimensional data representations found in foundation models.
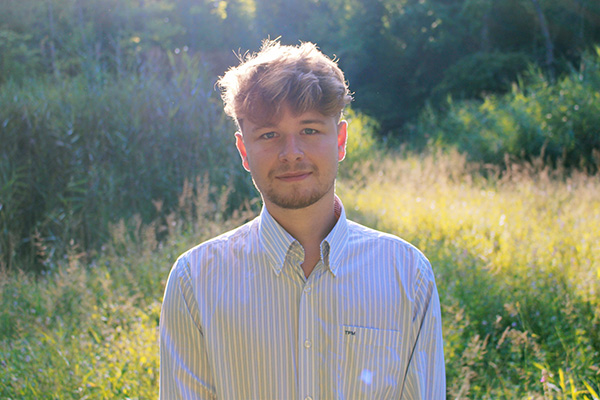
Florent Draye
Max Planck Institute for Intelligent SystemsSupervised by
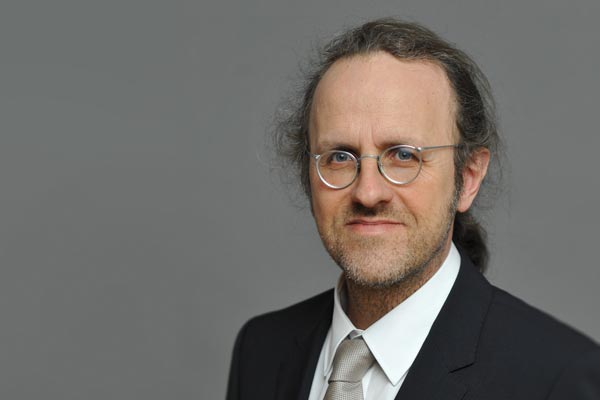
Bernhard Schölkopf
Informatics, Physics & MathematicsHector Fellow since 2018