Machine learning methods for gravitational-wave data analysis
Maximilian Dax – Hector Fellow Bernhard Schölkopf
The detection of gravitational waves (GWs) has opened a new window on the universe, through which we can study the physics of black-hole and neutron-star mergers. By analyzing GWs we can infer properties of the corresponding astrophysical systems. Current analysis methods are however too computationally expensive to deal with the growing amount of data. My research is thus concerned with the development of more efficient methods for the GW analysis using powerful machine learning methods.
The coalescences of binary systems of black holes or neutron stars emit gravitational waves (GWs) that encode information about the dynamics of the system. The detection of GWs therefore offers the exciting and unique opportunity to gain insight into these events. Current analysis methods are however slow and computationally expensive. This becomes increasingly problematic with the growing density of detected signals due to the continuous improvement of the detector sensitivity. More efficient analysis methods are therefore essential for the progress in gravitational physics. My research is concerned with the development of machine learning methods to accelerate the analysis of GWs.
My current focus is on inferring the parameters of astrophysical systems (e.g., the masses) from the GW data observed in detectors (‘strain’). This task can be interpreted as an inverse problem. In the forward direction the waveform for a given set of parameters can be simulated within the theory of general relativity. We are interested in the backward direction; given the measured strain data that contains the GW waveform and detector noise we estimate the parameters using powerful methods for simulation-based inference. Specifically, we train a deep neural network to model the so-called posterior distribution. Due to the amortization of computation for different events and the capability to generate posterior densities using only a forward pass through a neural network at inference time, our approach allows for rapid low-latency analysis of GWs.
Figure 1: Posterior densities of the masses of the binary-black hole system associated with the first ever detected gravitational wave GW150914. The result of the expensive standard method is shown in blue, the output of our neural density estimator in orange. The contours represent the 50% and 90% credible regions. Reprinted by permission of Stephen R. Green.
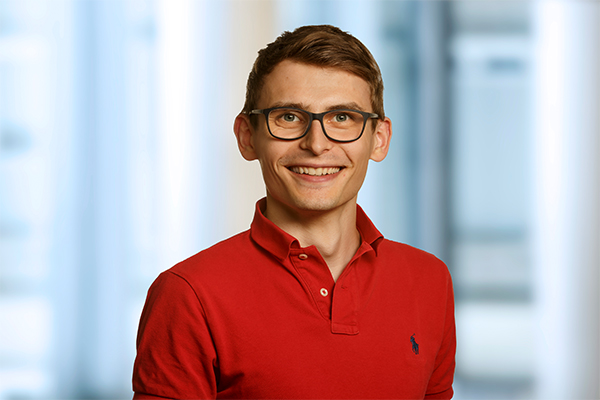
Maximilian Dax
Max Planck Institute for Intelligent SystemsSupervised by
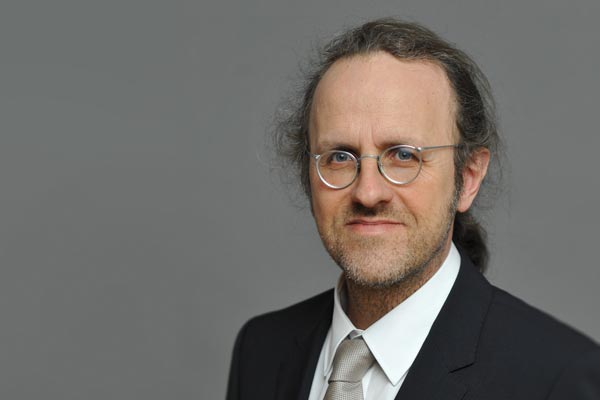
Bernhard Schölkopf
Informatics, Physics & MathematicsHector Fellow since 2018