Photonic Neuromorphic Circuits for Artificial Neural Networks
Martin Stecher — Hector Fellow Jürg Leuthold
We aim to develop artificial neural networks with brain-inspired circuits. Like in the brain, artificial neurons and synapses are built with novel memristors, arranged in a crossbar array. By combining these with ultra-fast photonics, we seek to improve signal processing and matrix-vector multiplications to address limitations from state-of-the-art architectures. This approach aims to advance computing solutions by reducing energy consumption, computational time, and system complexity.
We aim to develop hardware-based artificial neural networks using analogue circuits inspired by the human brain. Our approach relies on novel memristors that can mimic the behaviour of neurons and synapses by single-atom movement within them. Arranging these memristors in a crossbar-like structure allows us to create complex neuromorphic circuits for various applications. This new neuromorphic architecture requires specialised algorithms for in-memory computing, which will also be developed and researched.
The innovation in our approach lies in combining these analogue memristive circuits with ultra-fast integrated photonics, enabling us to handle data with greater speed and efficiency. Initially, we will focus on signal processing applications, where we aim to reduce system complexity and computational demands. As the project develops, we plan to advance toward more sophisticated circuits for general computing, addressing the energy consumption bottleneck associated with the conventional Von Neumann computer architecture.
The development of photonic-neuromorphic circuits for artificial neural networks marks a significant step toward rapid, low-energy, brain-inspired computing. This allows for revolutionising signal processing technology in the communication industry and developing a practical solution to reduce energy consumption through in-memory computing—a key challenge in scaling AI.
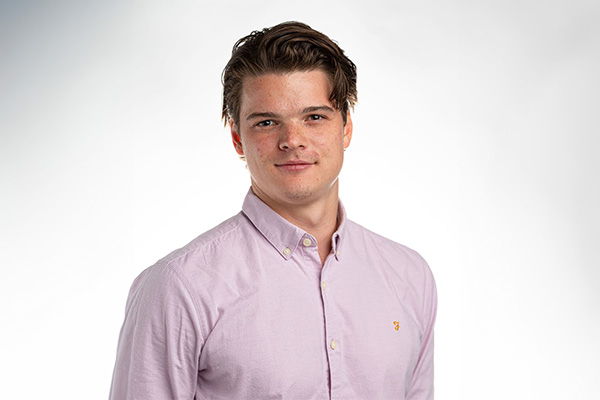
Martin Stecher
ETH ZürichSupervised by
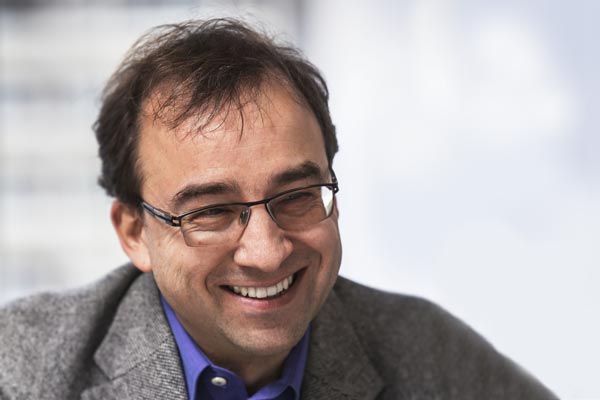
Jürg Leuthold
Physics & EngineeringHector Fellow since 2010